Artificial intelligence
Article curated by Holly Godwin
As our technology advances, are we edging closer to artificial intelligence, and what could be the consequences if we get there?
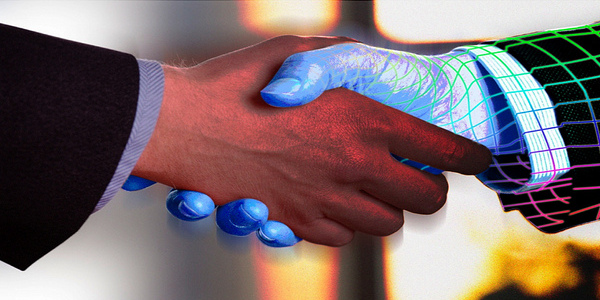
Nowadays, we use machines to assist us with our everyday lives, from kettles to laptops, and household robots, once the domain of science fiction, are already becoming a reality.
Some argue that this is an exciting advancement for human society, while others argue that it may have many negative connotations that we need to consider.
But what if machines are created that can exceed our capabilities?


Intelligence
If we’re to understand artificial intelligence, we first have to understand what we mean by “intelligence” at all – and it turns out that this is by no means a straightforward concept. Researchers trying to define intelligence in animals have had a go at defining criteria, including - tool use, tool making, and tool adaption - discrimination and comprehension of symbols and signs, alone or in sequences - ability to anticipate and plan for an event - play - understanding how head/eye movements relate to attention - ability to form complex social relationships - empathy - personality - recognising yourself in a mirror In other studies, intelligence is often defined as the ability to perform multiple cognitive tasks, rather than any one. We’re still not sure what the best intelligence criteria are, nor how to measure and weight it. In humans, factors like education and socioeconomic status affect performance, so measures are not necessarily a measure of “raw intelligence”, but also training: in fact, people are getting better and better at IQ tests as we learn how to perform them. We also don’t know how widely intelligence varies individual to individual, and therefore how wide a test sample is required to understand the breadth and depth of abilities. Differences in test scores can also reflect better level of motivation rather than ability, a measure which is not facile to standardise.



A lot has changed in our understanding of artificial intelligence and, in fact, intelligence itself. For example, we used to think that some tasks (e.g. image recognition, driving) required general intelligence – the ability to be smart across many domains and adapt intelligence to new circumstances. It turns out that this (and many other tasks) can be solved with narrow specialised intelligence. Research carried out on the muscle motion of the human eye, for example, has replicated its motions using soft actuators suitable for robotic applications. When paired with piezoelectric ceramic as the active material, this piezoelectric cellular actuator may be able to closely imitate the movements of the human eye, allowing robots to "see" and AI to adapt to human environments.



General intelligence
As it turns out, we don’t have any good examples of general artificial intelligence, and don't know what it would look like. It’s possible it doesn’t even exist. We certainly don’t know what general artificial intelligence might look like, and indeed it may look nothing like human general intelligence, and it’s structure may be very different from the human brain. The Turing Test was once thought of as a good test for general intelligence, but people are now creating machines that are being taught to pass the Turing Test – but do nothing else. This is a bit like the problem of students being taught how to pass exams rather than to understand the content.
Some think that generally intelligent AI may behave about rewards as humans do about emotions – prioritising their own above others’, whilst still recognising those of others exist. We have yet to find out.


When will AI be here?
Experts say this is inevitable: it’s hard to conceive of something you’re sure will happen, but will take more than 3 or 4 technology cycles to realise. And data that might help us make predictions (such as past examples) is limited. Indeed, expert models of when AI will be here exactly match those of the general public, i.e. your guess is probably just as good as an expert’s! Predictions made by both groups are over precise (because the error margins are huge) and overconfident about how soon we will get AI, both when made by scientists and by philosophers. We may, however, be able to improve our predictions in the future by reverse engineering them; that is, instead of asking when AI is most likely, ask if that prediction is wrong, what differences would you expect to see in the world? If you don’t see any differences, the prediction isn’t justified.


How would artificial intelligence change society?
If we had really intelligent AIs, what would the world look like? Doubtless, AIs would change stuff. In the future, blue collar crime may be easier to commit but also to detect, and war might take the form of blue collar crimes between states. Absolute poverty may be easily reduced, but relative poverty poses a harder problem – one that we don’t know whether AIs can fix. Medicine and medical treatments such as cognitive behavioural therapy are likely to become more technological, and the greatest challenges will be hacking and the problem of assigning culpability when the tech goes wrong (problems that also exist in other machine learning fields, such as self-driving cars).
Whatever happens, a utopic outcome is unlikely, as this assumes people want things that they should want – such as to contribute to communism. This is a hard world to imagine and so a hard problem for AI to solve, making the possibility of a dystopia seem more probable!
For example, it is very hard to programme a “safe” goal for AI. Imagine you successfully encoded an AI to prevent all human suffering… instantly it would kill all humans, as any other possibility would lead to more suffering. Imagine instead you encoded an AI to keep all humans safe and happy… it would sedate us all[1].
Does that mean AI will take over the world?
AI is an existential risk – extremely unlikely, but extremely devastating. This means that human brains are not great at processing understanding of the risk because it offends us to think the world could end by accident and not by some huge current political or moral issue.


At the moment, smaller issues in AI ethics include the lack of any ethics board or legislation around machine learning technology, and the discovery that machine learning technology are already racist and sexist, not being able to identify the gender of a black person, or understand a female voice – because of a bias in the input data. It’s possible that this could lead to all kinds of problems in the future: who takes the buck?


For now, however, there are still discrepancies between the abilities of machines and humans. While machines can be more efficient in some domains, such as mathematical processing, humans are far better equipped in many others, such as facial recognition.


Can artificial intelligence learn?
The capacity to learn would remove a significant constraint on AI abilities, and take a bold leap towards superintelligence. It has even been argued that AI can be human level and still superintelligent, because it would run many many times faster and learn many many times faster than we can.



Current research into machine learning focusses mainly on neural network programming. These artificial networks are statistical learning models, based on the neural networks found in the human brain.
An individual network consists of 10-30 stacked neurons. These neurons collaborate, so when an input stimulus (like an image) is detected, the first layer will communicate with the subsequent layer and so on until the final layer is reached. This final layer can then decide and produce a particular output, which serves as its ‘answer’, allowing us to assess how well the network recognised the input. Understanding how each layer analyses an image differently can be challenging, but we do know that each layer focusses on a progressively abstract features of the image. For example, one of the earlier layers may focus on edges while a later layer may focus on fine lines of detail. This is very similar to the process carried out in our brains as we break down features, shape, colour, et cetera.
Research to assess how efficient artificial intelligence was at recognising images and sound[2] has also looked at reversing the process: producing images (of bananas) from white noise. The interpretation was definitely recognisable as bananas, if bananas as viewed through a kaleidoscope. This ability was dubbed as ‘dreaming’ due to the slightly surreal effect, and illustrated that neurons designed to discriminate between images had acquired a lot of the information necessary to generate them. However, while the process is defined by well known mathematical models, we understand little of how and why it works.



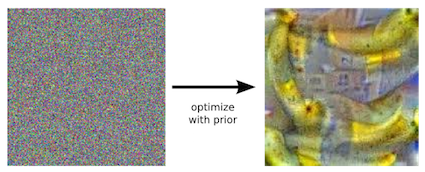
The problems with AI: Emotions
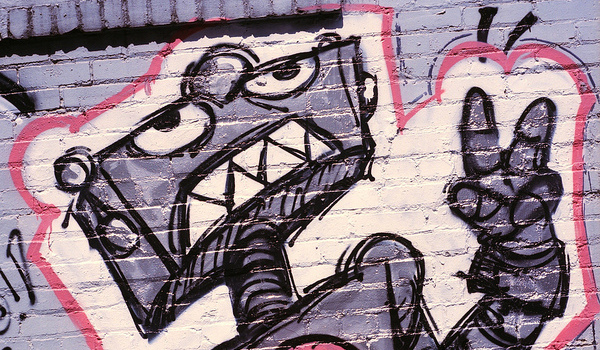
Decision-making and emotions are intricately linked. When facing difficult decisions with conflicting choices, often people are overwhelmed, and their cognitive processes alone can no longer determine the better choice. In order to come to a conclusion in these situations, it's been suggested we rely on ‘somatic markers’ to guide our decisions[3] – or the physical changes we undergo when we feel emotions.
These emotions go beyond the "primary" emotions of happy, sad, et cetera, including secondary emotions that are influenced by previous experiences and by culture. The somatic markers or physical sensations of emotion guide us in our decision-making, but we don't yet know if that is a good thing for difficult decisions or not.
So can AI make decisions without emotions? Are emotions purely obstacles to reason, or is intelligent thought the product of an interaction between emotion and reason?[4]. And is there an AI equivalent of emotions?



But are advances in this area going to merely produce better fakes, improvements on current simulations, or can we program a machine to feel? Many think this would entail a better understanding of human consciousness than currently exists, and opens the question as to whether manmade consciousness would be "good"?
Other scientists have made progress towards an AI equivalent to emotions through media such as the “mAIry’s Room” thought experiment. In this experiment, mAIry is confined to a grey room from birth and accesses the outside room through a black and white monitor. She is a brilliant scientist and has learnt all this is to learn about colour and perception. One day she experiences purple for the first time. The question asked by philosopher is, has she learnt anything? mAIry doesn't gain any extra explicit knowledge, but her brain has done something new in its processing.
This is known as TD-Lambda (temporal difference) learning: learning by the difference between a predicted reward and the actual reward. Where learning is the goal, AI can treat certain inputs as “special” or an “experience”, valuing them beyond their informational value. These inputs are thus AI “values”. This then allows smart AI to hold evolving values or ideas about what is “right”.
Scientists who are looking into these fields are employing state of the art learning techniques such as (deep) Q-learning and other model-free machine learning processes.
Learn more about Programming machines with emotions.



The problems with AI: Morality and consequences
AI with no sense of morality might perform destructive tasks, such as tiling the universe[4] – a thought experiment which describes a £5 note-making robot attempting to tile the entire universe with them, under the impression it was carrying out its given task. £5 notes would become valueless, and the AI's aggressive printing pursuit terrorism.
Equally, in the runaway train thought experiment, an AI working for the greater good would redirect a runaway train to kill one person instead of five, but many are divided on the morality of inaction[7]. Even more so when it comes to the hospital scenario, where five sickly patients are in need of different transplants and will die without them. An agent solely acting for the greater good should kill a healthy passer-by and use their organs to cure the patients, but few would do this, even many of those who would redirect the train! Would an AI acting for the greater good? It’s actually difficult to be sure. There are long term consequences to the hospital scenario: if people regularly get sacrificed for the greater good in hospitals, nobody would go, making the long-term utilitarian option not to sacrifice anybody. There’s still a lot we don’t know!
Another ethical problem is the dispersion of AI and its impact of social equality and war[4]. Additionally, should questions of ownership even be considered? If these beings rival, if not outdo, our own intelligence, should we have the right to claim ownership over them? Or is that enslavement?


The problems with AI: Social AI
The most dangerous AI are socially skilled AI.
If confined, AI are limited, but if set free and on the internet, they could rapidly acquire knowledge and pose a hazard, e.g. creating new kinds of weapons. It is thought that socially skilled AI will have the best chance of persuading us to let them out or sneaking past our defences. Once out, AI could be impossible to put back away, for example, by making itself indispensable to the economy or manipulating who ends up in charge of AI projects.
Experts are exploring ways to “keep AI in a box” – giving it enough information about the outside world to be useful, but limiting it enough (such as to yes/no answers) to mitigrate the risk.


.jpg)
The artificial intelligence ‘explosion’!
If superintelligent machines are an achievable possibility, they could act as a catalyst for an ‘AI explosion’[4].
A superintelligent machine with greater general intelligence than a human being (not purely domain intelligence) would be capable of building machines equally or more intelligent, starting a chain reaction and ‘AI explosion’! This argument suggests one way we could lose control over machines.


Going out with a whimper
Without an "intelligence explosion", smart AIs can still be a problem. For example, if our aim is to prevent crime, and we assess our success by measuring the number of reported crimes, the easiest way to maximise success and reduce the number of reported crimes is by making it harder to report crimes or introducing negative consequences to those who do. Neither of these would address the actual problem of reducing crime, but they would address our measurement of it. (This may be better known as the “Jobcentre problem”, where Jobcentres success is measured not by getting people jobs, but by getting them off the dole…). AIs might amplify institutional problems like this because they can be programmed to complete measurable tasks and can model strategies to identify the ones which affect our measured outcomes most. You can also gain support for your idea either by improving it, or by reaching more people.
Eventually, where value is measured in profit, profit will be obtained at a cost in value (e.g. companies stealing from consumers). This AI end game, where they effectively take over the system and humans become unable to compete with its trial-and-error improved systematic manipulation and deception is called “going out with a whimper.”


The questions related to this field are numerous. It is a topic at the frontier of science that is developing rapidly, and the once futuristic fiction is now looking more and more possible. While the human race always has a drive to pursue knowledge, in a situation with so many possible outcomes, maybe we should exercise caution. This article was supported with advice and information from Dr Stuart Armstrong, Future of Humanity Institute, University of Oxford.
This article was written by the Things We Don’t Know editorial team, with contributions from Ginny Smith, Johanna Blee, Rowena Fletcher-Wood, and Holly Godwin.
This article was first published on 2015-08-27 and was last updated on 2020-06-02.
References
why don’t all references have links?
[1] Armstrong, Stuart. 2014. Smarter Than Us: The Rise of Machine Intelligence. Berkeley, CA: Machine Intelligence Research Institute.
[2] Mordvintsev, A., Olah, C., Tyka, M. ‘Inceptionism: Going Deeper into Neural Networks’ Google Research Blog, 2015.
[3] Damasio, A., Everitt, B., Bishop, D. ‘The Somatic Marker Hypothesis and the Possible Functions of the Prefrontal Cortex’ Royal Society, 1996.
[4] Evans, D. ‘The AI Swindle’ SCL Technology Law Futures Conference, 2015.
[5] Evans, D. ‘Can robots have emotions?’ School of Informatics Paper, University of Edinburgh, 2004.
[6] Bostrom, N., Yudkowsky, E. ‘The Ethics of Artificial Intelligence’ Cambridge Handbook of Artificial Intelligence, Cambridge University Press, 2011.
[7] Kant, I. ‘Grounding for the Metaphysics of Morals’ (1785) Hackett, 3rd ed. p.3-, 1993.
Recent artificial intelligence News
Get customised news updates on your homepage by subscribing to articles